Diese Case Study ist auch auf Deutsch verfügbar
At a glance
- Analysis of the historical, multidimensional data and visualization of the special features
- Development of a methodology to increase data quality
- Full implementation of a deep learning model including model design, training processes, and inference
- Visualization of the quality of the forecast data for quality control of the model and also of the original data
- Integration of the data in the corresponding systems
- Deployment of the system in multiple settings, including production
In order to determine the maximum load of a concrete support – for example for a multistory building – and to select the correct type of support, a whole array of parameters are important. These include the weight to be supported, the type of concrete, the type and amount of reinforcing steel, the requirements on the fire resistance rating, the length of the supports, and the location within the construction. As many as 30 different parameters must be considered in order to fully describe the support.
SACAC previously calculated the load of the supports for the purposes of tender preparation by means of the finite element method (FEM). This is a mathematical model for complex physical tasks in which the component is broken down into much smaller tetrahedrons. This is a very laborious process, as the software is licensed and cannot be automated or integrated in any way. The FEM therefore has to be manually undertaken and the calculations done by hand.
The value of good values
Fortunately, SACAC had always made a point of comprehensively collecting and saving all load values that had been calculated. By no means a common (or a particularly popular) procedure, as the transferring of the values meant a significant amount of additional work for the members of staff. But it meant that previously undertaken calculations could be reused by employees for similar supports. And this policy was also very foresighted in respect of machine learning. Because over time, countless values had been recorded by the SACAC employees.
The potential of these values was enormous. But in order for them to be used for the preparation of tenders, a solution was required that could automatically calculate large quantities of such values. The INNOQ team therefore came up with the idea of training a deep learning system to forecast the load values at a level of quality good enough for use in tender preparation. As a result, the complex calculations with the FEM would no longer be needed, either in the sales process or in the preparation of tenders.
INNOQ supports us as a partner not only in the technical implementation but also in the strategic digitalization of our business model.
Lukas UntereggerHead of Engineering, SACAC
As part of the project, a complete machine learning pipeline was implemented, from preparation of the data and the training of the model to deployment in the production. Now when preparing a tender, thousands of specifications can automatically be calculated. The deep learning model is able to calculate load values on this basis – with impressive accuracy. These calculations have on average a deviation of only 5%. In 95% of all cases, the deviation is 10% or less. And the accuracy is increasing even further over time.
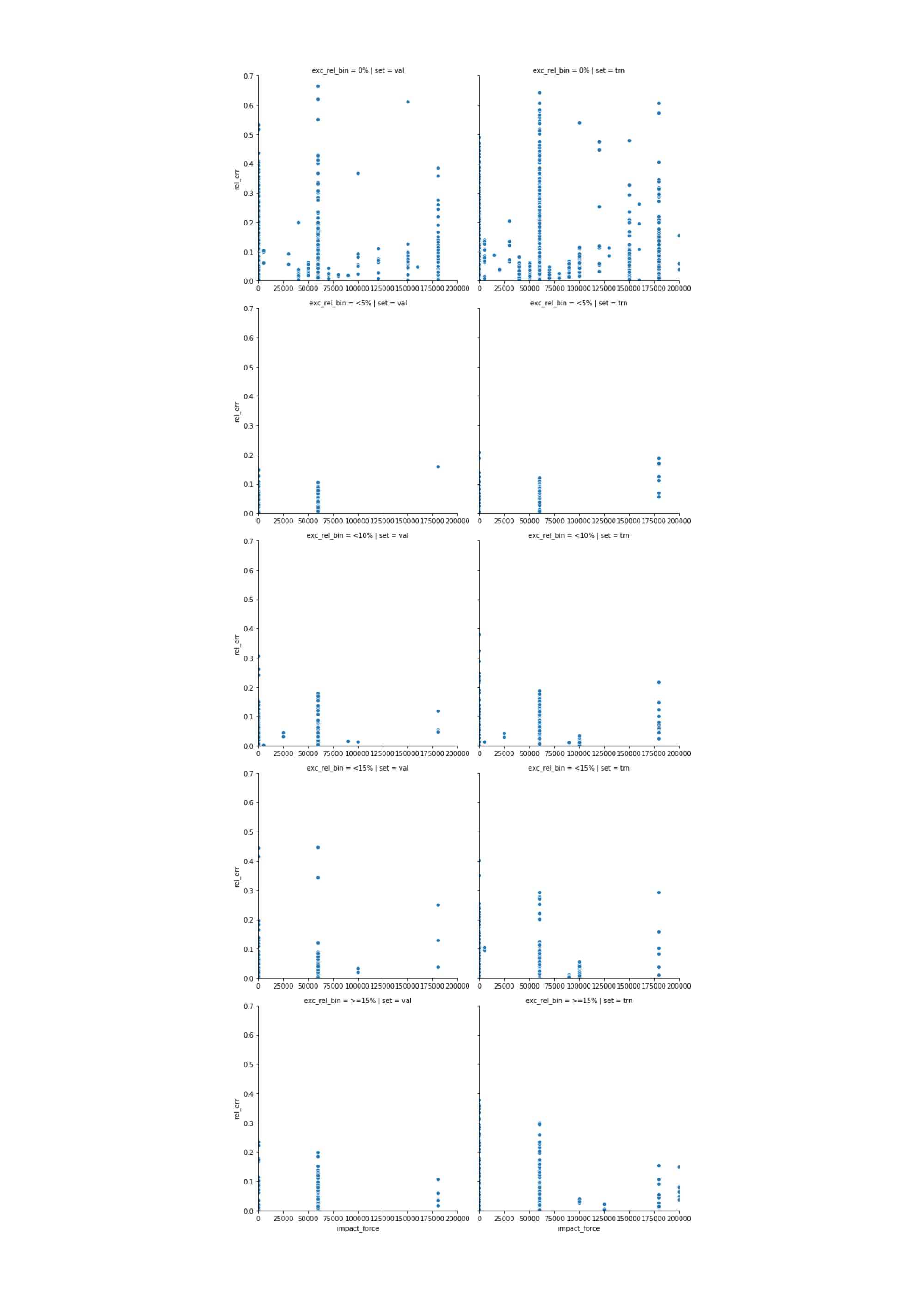
In order to evaluate the deep learning system, a customized metric was developed with the client. Because the assessment of the quality of a deep learning system has proven to be nontrivial in practice. Developing such a new system requires new versions to be compared with previous versions and deciding which is better. Often, new versions are better in some aspects but perhaps worse in others. Ensuring a balanced evaluation of these many differences is an important task, one that the subject experts at SACAC and the machine learning experts at INNOQ could only achieve together.
A question of competitive advantage
There were previously two problems with the preparation of tenders that resulted above all in an economic risk. If a profile was selected for the tender that was too large for the specific case and therefore too expensive, there was a high risk of losing the contract. Conversely, if an overly weak, but as a result cheaper, support was offered, SACAC would suffer losses in the undertaking of the contract as more expensive supports were needed than originally calculated. Today however, the software provides suggestions on the basis of the stipulated parameters that are so accurate that miscalculations can largely be avoided. As a result, the commercial risks are minimized.
An example
According to the plans, a support is needed in a specific position in a multistory building that is 3 meters long and must carry 1,500 kilonewtons. The engineer decided for design reasons on a round support with a diameter of 200 millimeters.
On the basis of this data and the stipulated parameters related to the load-bearing capacity, for example the eccentricity and concrete strength, the software presents a list of suitable support profiles. It also marks the supports that have previously been calculated in detail by a SACAC engineer.
The show-stopper: the values in the list obtained through machine learning present a very accurate estimate of the load. The experts can therefore see at a glance which supports are ideal and which price is appropriate for the offer. This not only saves the employees of SACAC a lot of time, but also results in a more precise offer. In this example, the possible price spanned from 35 to 88 Swiss francs per meter, which makes a big difference on the cost of the construction project as a whole. Now the best-value solution for the client can be found and a competitive offer submitted.
Of course SACAC doesn’t blindly rely on artificial intelligence. The estimations generated by the software only serve as a basis for the offer phase. If the offer is accepted, the calculation of the supports is carefully inspected by an engineer before production starts.