In this article, I want to show how data products for a data mesh can be created with the help of Terraform. The presented work should be a starting point for both data people to get into Data Mesh and for the open-source community to develop collaboratively helpful tools based on real customer needs within the industry.
All examples and the developed modules use AWS and corresponding services. However, there are a lot more ways to implement a data mesh architecture. [1]
Let’s start with a brief overview about data mesh, data products and Terraform.
What is Data Mesh?
Data Mesh is a new sociotechnical approach to decentralized data architecture with the goal of deriving value from data even in large enterprises. It is based on four principles:
-
Domain ownership
Domain teams take responsibility for their operational and analytical data -
Data as a product
Applies product thinking philosophy to domain teams and their operational and analytical data -
Self-serve data infrastructure platform
Apply platform thinking to data infrastructure enabled by a dedicated data platform team -
Federated governance
Achieves interoperability of all data products through standardization
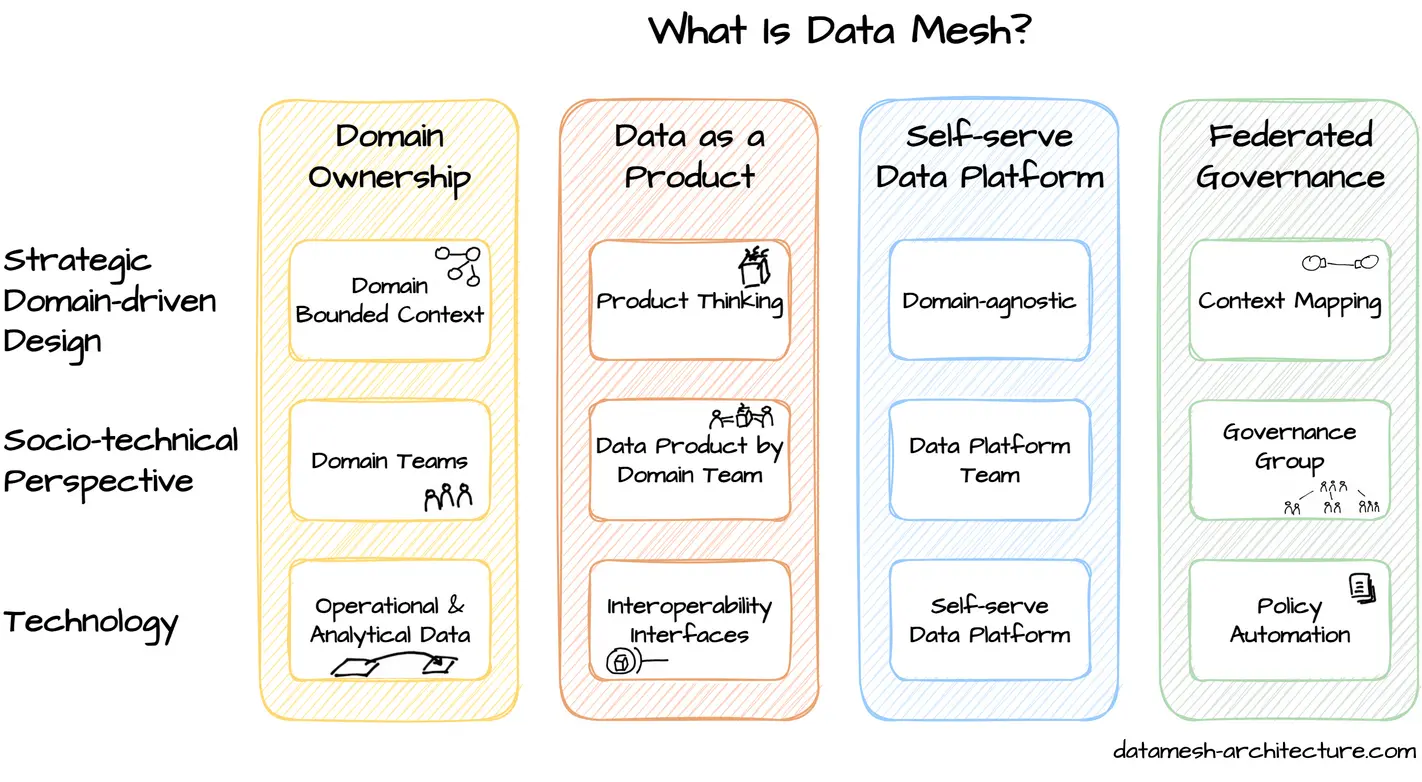
If you have further interest to learn about Data Mesh, I’d recommend the following resources:
- My colleague Jochen Christ wrote a great article about the idea and the main concepts behind Data Mesh
- My colleague Daniel Lauxtermann wrote an article to answer the question, why your (software) team need data products
- Also, I want to mention the original source of the idea of Data Mesh: The book «Data Mesh: Delivering Data-Driven Value at Scale» from Zhamak Dehghani
- There is also a German translation «Data Mesh: Eine dezentrale Datenarchitektur entwerfen» from my colleagues Jochen Christ and Simon Harrer
One of the core components of Data Mesh is a Data Product. In the next section, we’ll dive into what a data product is.
What is a data product?
A data product is an autonomous technical component that contains all data, code, and interfaces to serve the domain team’s or other teams' analytical data needs. You can think of a microservice, but for analytical data.
Data products connect to sources, such as operational systems or other data products, and perform data transformation. Data products can produce analytical data in different formats.
For a better understanding and to support the design process of data products, my colleagues propose the so-called «Data Product Canvas», inspired by the Bounded Context Canvas from Domain-Driven Design:
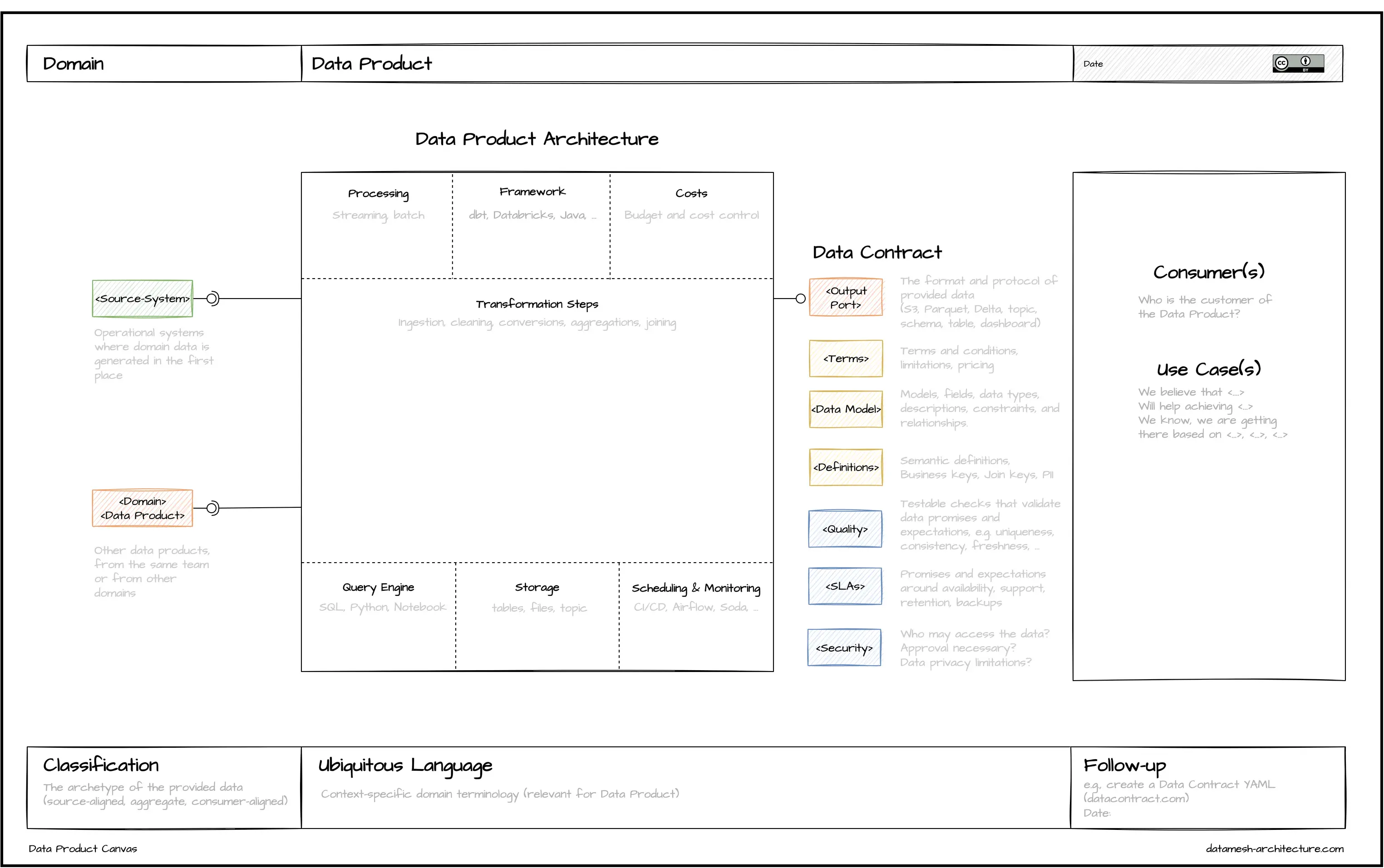
Looking at the canvas and the structure of a data product, it has
- one or more input ports (where data should be read from)
- some transform logic (what should be done with the input data) and
- an output port (the result of the transform logic, including data format and protocol).
Also, a data product should expose metadata (e.g. information about the ownership) and details about observability (e.g. quality metrics) through separate endpoints.
Since we now know what a data product is and how it should look like, we can start to create one on AWS.
Data products on AWS
Fortunately, my colleagues already created a recommendation which AWS services can be used, based on their practical experience:
-
AWS Athena
Execute queries with SQL or Python on data stored in e.g. operational databases or data warehouses -
AWS Glue
Data integration service to simplify e.g. discovery or preparation of data from multiple sources -
AWS S3
Object storage service -
AWS Lambda
Compute service that lets you run code
Bringing those four services and the concept of a data product together, we could use AWS S3 (more specifically a S3 bucket) as an input port. We can also store the output data of our transformation in a S3 bucket. To be able to describe the input and output data, it’s possible to add schemas (e.g. JSON Schema) and corresponding data tables to AWS Glue. What’s left is the actual execution of the data transformation. This can be achieved with a small application written for AWS Lambda, which calls AWS Athena to execute a query on a specified data source.
As you might guess, we now have reached the point to move over to AWS and to create the described resources. Basically, there are two possible ways of doing so:
I see a few down-sides with this approach:
- Even if we know which services to use, we have to click through the web interface or use the CLI to provision all necessary services. Furthermore, we have to know how we have to connect the services on a technical-level. So far, I have totally ignored the security aspect as well.
- Let’s ignore the previous bullet point and say that you were able to provision and configure the necessary services: What do you hand-over to another interest who wants to create a data product? A text document or a link to a Confluence page with a step-by-step guide? Such guides are might helpful and can be used for documentation purposes. However, when it comes to manual procedures, Tip 95 from the book «The Pragmatic Programmer»[2] comes to my mind:
Don’t Use Manual Procedures
As stated in the book, «people just aren’t as repeatable as computers are».
Fortunately, software developers already solved these downsides, which brings us to the next building block within this article: Terraform.
What is Terraform?
Terraform is an infrastructure-as-code tool, which enables developers (or in our case the domain teams) to automate provisioning, compliance, and management of any cloud. It uses a declarative configuration language, «HashiCorp Configuration Language (HCL)». The HCL definitions are stored in .tf
files and can therefore be versioned within the domain team’s code repository.
The following example shows how an AWS S3 bucket can be provisioned on AWS with Terraform:
resource "aws_s3_bucket" "my_aws_s3_bucket" {
bucket = "my-aws-s3-bucket"
tags = {
Name = "My bucket"
Environment = "Dev"
}
}
Terraform takes care of comparing all locally defined resources (the desired state) with all existing resources on the remote side (the actual state, in our example on AWS). In case of a difference between the desired and actual state, Terraform will apply all necessary changes (CRUD) to match the desired state.
Another important concept are Terraform Modules:
Modules are containers for multiple resources that are used together. A module consists of a collection of .tf and/or .tf.json files kept together in a directory. Modules are the main way to package and reuse resource configurations with Terraform.
With Terraform modules it’s possible to organize, encapsulate and re-use (complex) configurations. This can reduce errors and will improve the consistency when writing new modules.
The typical structure of a Terraform module is the following:
.
├── LICENSE
├── README.md
├── variables.tf
├── main.tf
├── outputs.tf
The files LICENSE
and README.md
are self-explanatory. variables.tf
is used to specify the inputs of the Terraform module. Each input has a data type such as string, list or object. In main.tf
the actual module is defined, e.g. which services should be provisioned based on the input defined in variable.tf
. Within the last file outputs.tf
all outgoing data of the module can be defined. This is useful when connecting multiple Terraform modules with each other, where the «succeeding» modules should use an output value of a «previous» module.
Within a module, it’s also possible to define further *.tf
files to organize and structure a configuration. All resources in the same folder can be referenced:
resource "aws_s3_bucket" "my_bucket" {
bucket = "my-aws-s3-bucket"
}
module "my_terraform_module" {
bucket_name = aws_s3_bucket.my_bucket.bucket # Access name of the S3 bucket
}
Terraform should enable us to create one or more modules, which contain the steps to provision necessary resources and their interconnections, including rights management. Afterwards, arbitrary domain teams can use those modules and setup data products in a repeatable (and hopefully less error-prone) way.
Write a module for Terraform
As mentioned in the previous section, Terraform should provide the necessary features to create data products in a declarative and re-usable way. First, we’re faced with the question of how the module should look like from a user-perspective.
How should the module look like from a user-perspective?
A good starting point is to have again a look at the Data Product Canvas described under «What is a data product?». Besides input and output ports, we need a transformation part (e.g. an SQL query). It would also be good to be able to assign a name and the corresponding domain to the data product.
Transferred to Terraform, this would look like the following:
module "data_product" {
domain = "<domain_name>"
name = "<product_name>"
input = "<some_input>"
transform = "<some_transform>"
output = "<some_output>"
}
What we’ve missed in our code snippet from the Data Product Canvas are the other endpoints «Metadata» and «Observability». In terms of software development, both can be described as machine-readable endpoints (e.g. REST-endpoints), which can be consumed by other services (e.g. a catalog which displays all existing data products). These endpoints could also be used as inputs for other data products. However, from a user-perspective this is nothing we want to define. The data product should «just» make available these endpoints for us.
Based on our considerations, we can now go one step deeper and think about the internal structure of our Terraform module.
The inner core
As mentioned earlier, Terraform allows us to organize the module code in different files. Since we already know which AWS services we need and how the parts of a data product should be named, the following structure should sound familiar:
.
├── main.tf
├── variables.tf
├── s3_bucket.tf
├── data_catalog.tf
├── transform.tf
├── endpoint.tf
-
variables.tf
defines all necessary placeholders (from a user-perspective), e.g. «domain», «name» and «input» -
s3_bucket.tf
contains all statements for the provisioning of the AWS S3 bucket (output) -
data_catalog.tf
takes care of provisioning the AWS Glue resources (e.g. data schema) -
transform.tf
setups an AWS Lambda services, which calls AWS Athena with the specified query (transform) -
endpoint.tf
provisions necessary endpoints for metadata and observability
The following picture shows a graphical representation of all used services and their interconnections:
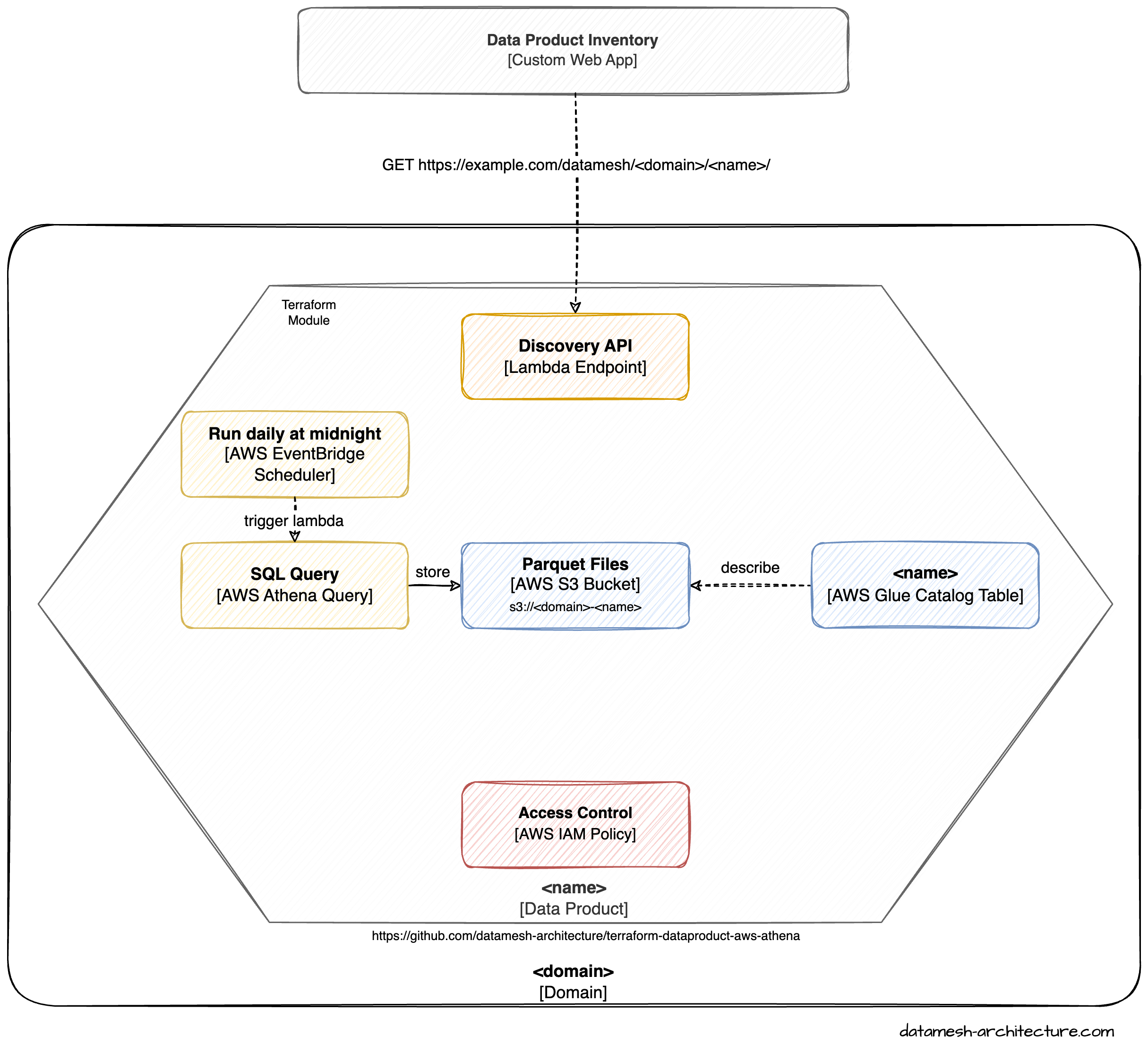
The entire code of the current state of the module can be found in the repository on GitHub.
Let’s create a data product
To get an idea of how the previous work can be used to create data products, let’s start with a real world example.
An online retailer with local stores wants to identify so-called «shelf warmers»[3]. All stock changes will be published via Kafka. All messages will be stored within an AWS S3 bucket. The data has the following structure:
{
"location": "042",
"article": "b0cdf759-ec06-41a3-b253-7ac907fea97",
"quantity": 5,
"timestamp": "2022-12-01T10:42:00Z"
}
The data engineer of the domain team has already written an SQL query to identify all shelf warmers within the stored data. With our previous work, we can create the following data product:
module shelf_warmers {
source = "[email protected]:datamesh-architecture/terraform-dataproduct-aws-athena.git"
domain = "fulfillment"
name = "shelf_warmers"
input = [ "s3://fulfillment-stock-updated" ]
transform = "sql/transform.sql"
output = {
schema = "schema/shelf_warmers.schema.json"
format = "PARQUET"
}
}
The module «shelf_warmers» references the git repository of our developed Terraform module. All listed variables are taken from our previously defined variables.tf
file. The input for our data product is a link to an existing AWS S3 bucket. Transform points to the SQL file written by our data engineer. Output describes that we’re producing Parquet files based on the referenced JSON schema.
All we have to do now (besides adding our credentials for AWS, which I have omitted here) is to start provisioning the necessary resources with the corresponding Terraform command:
terraform apply
This will create a long list of resources which needs to be created by Terraform. The following snapshot shows only a small excerpt of the output:
dataproduct-shelf_warmers % terraform apply
**module.shelf_warmers.data.aws_iam_policy_document.allow_athena: Reading...**
**module.shelf_warmers.data.aws_iam_policy_document.allow_logging: Reading...**
**module.shelf_warmers.data.aws_iam_policy_document.lambda_assume: Reading...**
**module.shelf_warmers.data.aws_iam_policy_document.allow_glue: Reading...**
**module.shelf_warmers.data.aws_iam_policy_document.allow_s3_input: Reading...**
**module.shelf_warmers.data.aws_iam_policy_document.lambda_assume: Read complete after 0s [id=3693445097]**
**module.shelf_warmers.data.aws_iam_policy_document.allow_logging: Read complete after 0s [id=3362990530]**
**module.shelf_warmers.data.aws_iam_policy_document.allow_athena: Read complete after 0s [id=1490858917]**
**module.shelf_warmers.data.aws_iam_policy_document.allow_glue: Read complete after 0s [id=2747066667]**
**module.shelf_warmers.data.aws_iam_policy_document.allow_s3_input: Read complete after 0s [id=2375223563]**
Terraform used the selected providers to generate the following execution plan. Resource actions are indicated with the following symbols:
+ create
<= read (data resources)
Terraform will perform the following actions:
# module.shelf_warmers.data.archive_file.archive_info_to_s3 will be read during apply
# (depends on a resource or a module with changes pending)
...
Plan: 30 to add, 0 to change, 0 to destroy.
Do you want to perform these actions?
Terraform will perform the actions described above.
Only 'yes' will be accepted to approve.
Enter a value:
After entering yes
the process continues and all necessary services will be provisioned.
Via the HTTP REST endpoint, it’s possible to retrieve information about the data product:
GET https://3jopsshxxc.execute-api.eu-central-1.amazonaws.com/prod/
Content-Type: application/json
{
"domain": "fulfillment",
"name": "shelf_warmers",
"output": {
"location": "arn:aws:s3:::fulfillment-shelf-warmers/output/data/"
}
}
Conclusion
In this article, I presented the current state of our work to create data products for a Data Mesh on AWS. To provision the necessary resources and create a usable interface for people without a software development background, I’ve used Terraform (Modules). The results of the work can be found as open-source modules on GitHub.
Additional notes
- In the small example, I assumed, that the data related to stock changes is already present on AWS S3. However, I have also written another Terraform module, which transfers Kafka messages to an AWS S3 bucket.
- This article does not show the entire functionality of the developed Terraform modules. For further information, have a look at the linked GitHub repositories or get directly in touch with us.
- The current state does not have all features mentioned by Zhamak Dehghani in her book Data Mesh. Instead, it’s a starting point for a collaborative, open-source development based on real customer needs within the industry.
Links and references
- Terraform Module «Data Product AWS Athena»
- Terraform Module «Data Product Confluent Kafka to S3»
- Example from this article
- Data Mesh Architecture
- Data Mesh Training @ socreatory
Mehr zum Thema Data Mesh? Wir bieten ein 2-Tages-Training an.